Published
By
Share this page
In the vast realm of artificial intelligence, few fields have captivated our imagination and pushed the boundaries of possibility quite like computer vision. At the core of this domain of research and innovation lies the ambition to empower technologies for real-world vision-based systems, enabling machines to take in and respond to visual stimuli with unparalleled precision and sophistication. Through the combination of AI, deep learning, and vast amounts of data, computer vision has made great strides in recent years, catapulting us into an era in which the seemingly impossible becomes achievable.
The Computer Vision and Pattern Recognition (opens in new tab) (CVPR) 2023, held June 10 through June 22, is a widely recognized event that brings together leading experts in the field of computer vision. It serves as a platform for showcasing some of the most compelling and innovative work in this domain.
The contributions presented by Microsoft researchers and their collaborators at this year’s CVPR cover a wide spectrum of research endeavors. From generative models and network pretraining to sign language understanding and neural video codecs, these cutting-edge advancements underscore the evolving capabilities of systems to analyze and extract valuable insights from visual data.
Here are some of the highlights (see below for a list of published papers and their authors):
The paper, “Image as a Foreign Language: BEiT Pretraining for Vision and Vision-Language Tasks” lies at the intersection of vision, language, and multimodal pretraining. To learn from these different forms of data, we present a general-purpose foundational model that treats images as a “foreign language.” The data from different modalities are encoded with Multiway Transformers, a modular architecture that enables modality-specific encoding and deep fusion. The model is pretrained on images, text, and image-text pairs in a way that generalizes the masked language modeling approach to different modalities. By substantially scaling the model and data, we found that these advances in foundational architecture and pretraining lead to excellent transfer performance over a variety of vision and vision-language tasks, including object detection, semantic segmentation, image classification, visual reasoning, visual question answering, image captioning, and cross-modal image retrieval.
The strength of large language models stems from their ability to leverage unlabeled training data on a massive scale. By using this data, these models acquire a broad understanding of language, enhance their generalization abilities, and improve their performance across a wide range of language-related tasks. Inspired by this achievement, our research focuses on the possibilities of scaling training data for large vision models. In the paper “On Data Scaling in Masked Image Modeling,” we explore the effects of data scaling on large vision models that are pretrained through masked image modeling. Through extensive investigation, we discovered that masked image modeling in large vision models requires large-scale data for effective pretraining. However, unlike large language models, large vision models cannot benefit from more data in a non-overfitting scenario. These findings deepen our understanding of masked image modeling and may pave the way for future advancements in large-scale vision models.
In the world of image generation, incredible strides have been made in transforming text descriptions into stunning visuals. The rise of DALL-E and diffusion models has brought these cutting-edge tools into the hands of everyday users. In the paper “RODIN: A Generative Model for Sculpting 3D Digital Avatars Using Diffusion,” we expand on this innovation by introducing the power of diffusion to 3D avatar generation. To do this, it is necessary to transfer diffusion from 2D to 3D. However, transferring diffusion from 2D to 3D is a significant challenge due to the prohibitive memory and processing costs for producing high-quality results with rich details in 3D. We overcome this problem by proposing the roll-out diffusion network (RODIN), which unrolls a 3D neural radiance field into a single 2D feature plane and performs 3D-aware diffusion on it. Supported by other technical contributions, including latent conditioning to promote global coherence and hierarchical synthesis to further enhance details, RODIN significantly accelerates the otherwise tedious 3D modeling process and opens new opportunities for 3D artists.
Spotlight: Event Series
Join us for a continuous exchange of ideas about research in the era of general AI. Watch Episodes 1 & 2 on-demand.
Microsoft papers published at CVPR 2023 with their authors:
Distinguished Scientist
Senior Principal Research Manager
Follow us:
Share this page:
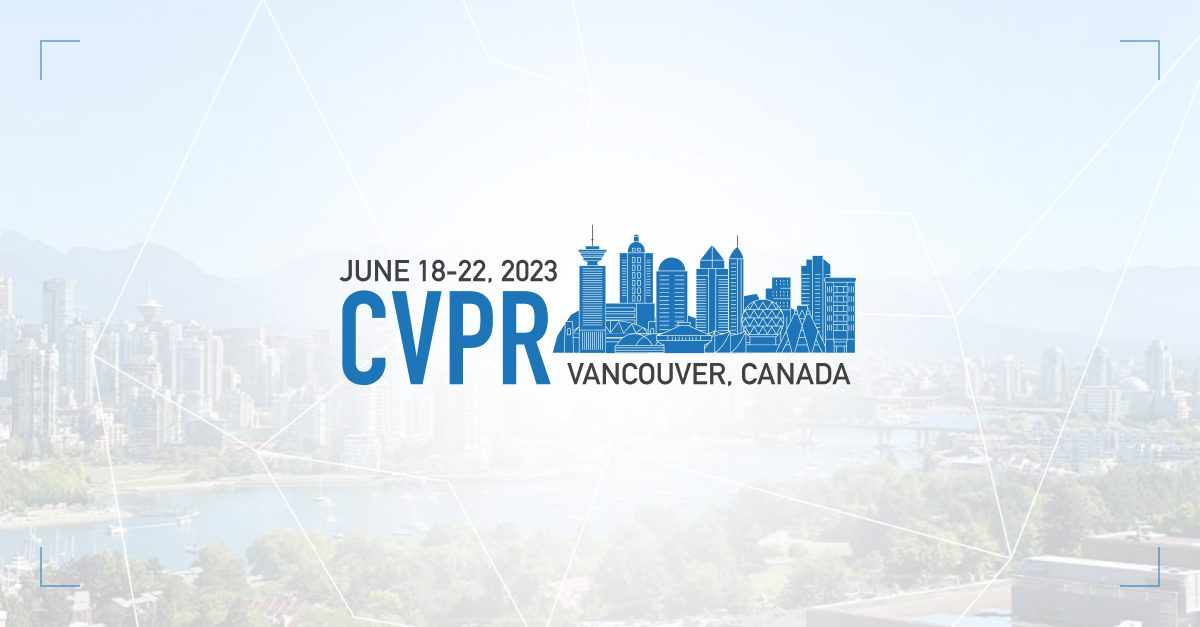
Leave a Reply